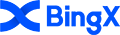
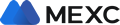
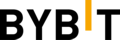
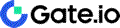
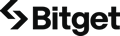
SingularityNET to Hold Live Stream on YouTube on May 7th
SingularityNET will host two mini-AMA series on YouTube on May 7th at 5 pm UTC. The series discusses the latest advancements in developing a unified experiential learning component for OpenCog Hyperon, their framework for Artificial General Intelligence (AGI) at the human level and beyond.
The first session will cover the implementation of the Non-Axiomatic Reasoning System (NARS) in OpenCog Hyperon’s MeTTa language cognitive computations and the integration of the Autonomous Intelligent Reinforcement Interpreted Symbolism (AIRIS) causality-based learning AI into Hyperon. The second session will focus on recreating experiential learning in Hyperon using the Rational OpenCog Controlled Agent (ROCCA) and porting fundamental components ROCCA requires from OpenCog classic to Hyperon, including forward and backward chaining, Probabilistic Logic Networks (PLN), and pattern mining.
What is AMA?
An AMA (ask me anything) is a usually online informal interactive meeting where participants are free to ask the guest questions and get answers in real time.
@
Session 1
- The implementation of NARS (Non-Axiomatic Reasoning System) in OpenCog Hyperon’s MeTTa language cognitive computations;
- Integrating the AIRIS (Autonomous Intelligent Reinforcement Interpreted Symbolism) causality-based learning AI into Hyperon.
Session 2
- Recreating experiential learning in Hyperon using ROCCA (Rational OpenCog Controlled Agent);
- Porting fundamental components ROCCA requires from OpenCog classic to Hyperon, including forward and backward chaining, PLN (Probabilistic Logic Networks), and pattern mining.
These advancements are part of our ongoing initiative to consolidate the strengths of several systems —ROCCA, NARS, OpenPsi, and AIRIS— to create a unified experiential learning component for Hyperon. This approach will allow AI models to:
- Develop a goal-independent understanding of their environment through causal knowledge gained from planned and spontaneous interactions;
- Explore their environment with increased efficiency using a curiosity model that prioritizes situations with high uncertainty, challenging their existing causal knowledge.
Our preliminary findings indicate that this approach surpasses common Reinforcement Learning techniques in terms of data efficiency by orders of magnitude.
To learn more, set your reminder for the livestream now on your preferred platform:
- YouTube: https://t.co/66Xm1SpDC8
- LinkedIn: https://t.co/JEwoyT7Z9R
- X: SingularityNET